Our people
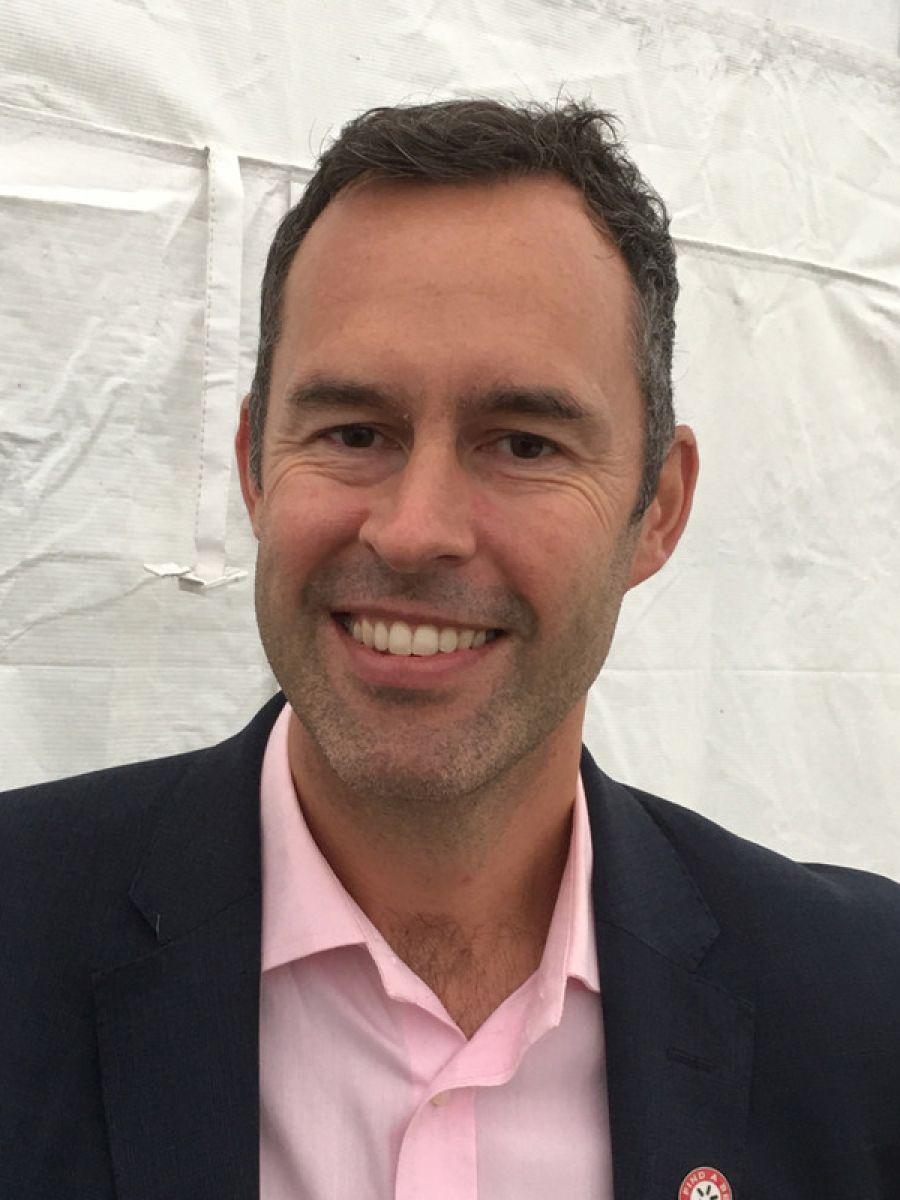
Professor Tom Scott
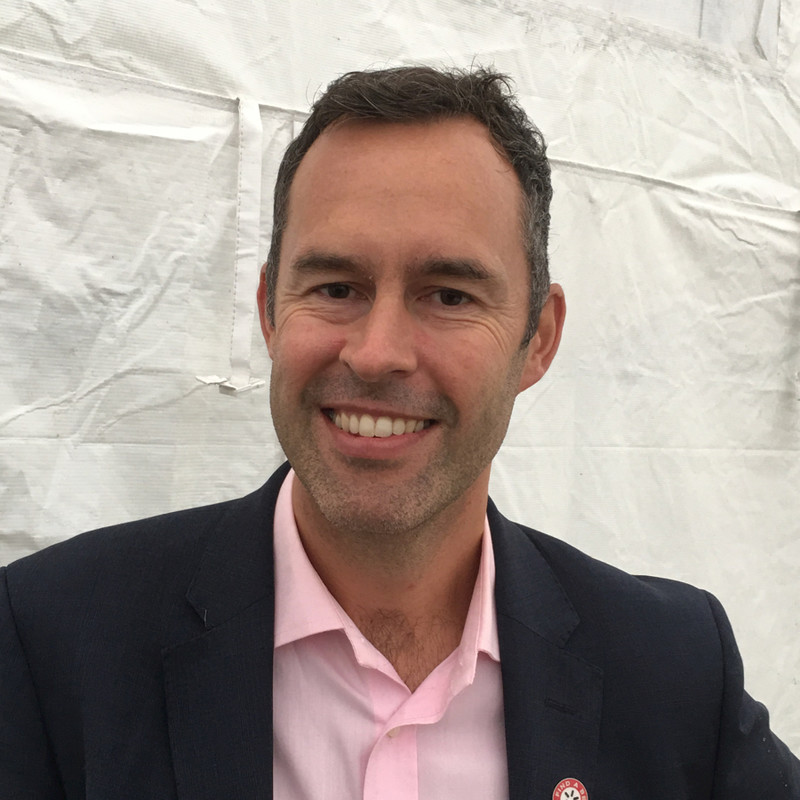
Professor Tom Scott
Professor Scott’s research is based around ageing, corrosion and characterisation of radioactive materials in engineered and environmental systems. He is the academic lead for the Sellafield UK Centre of Expertise for Uranium and Reactive Metals. Working with Sellafield he has successfully developed and deployed two novel radiation detection technologies in the past 5 years, including the Advanced Airborne Radiation Monitoring (AARM) system which won the 2014 RAEng ERA award for innovation.

Professor Simon Middleburgh
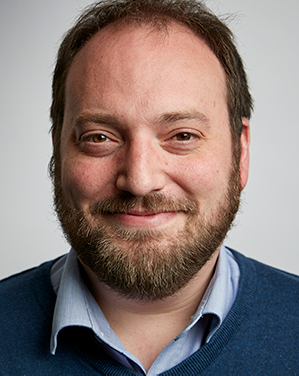
Professor Simon Middleburgh
Simon was appointed to Bangor University’s Nuclear Futures Institute in March 2018. His research is focused on developing new nuclear materials, investigating material behaviour in extreme environments (including nuclear and aerospace) and combining materials modelling techniques with experimental methods. He is a Fellow of the Institute of Materials, Minerals and Mining (FIMMM) and Member of the Institute of Physics (MInstP).
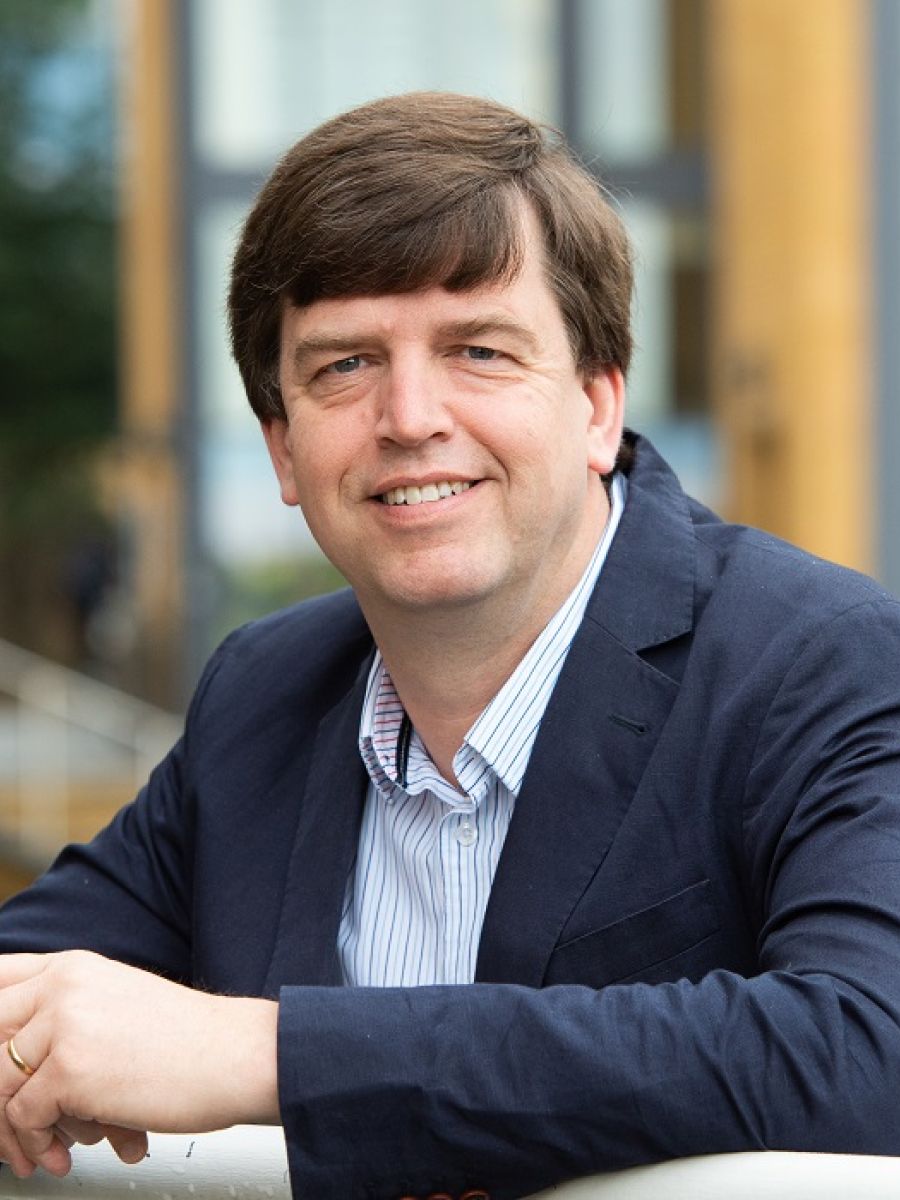
Professor Paul Sellin
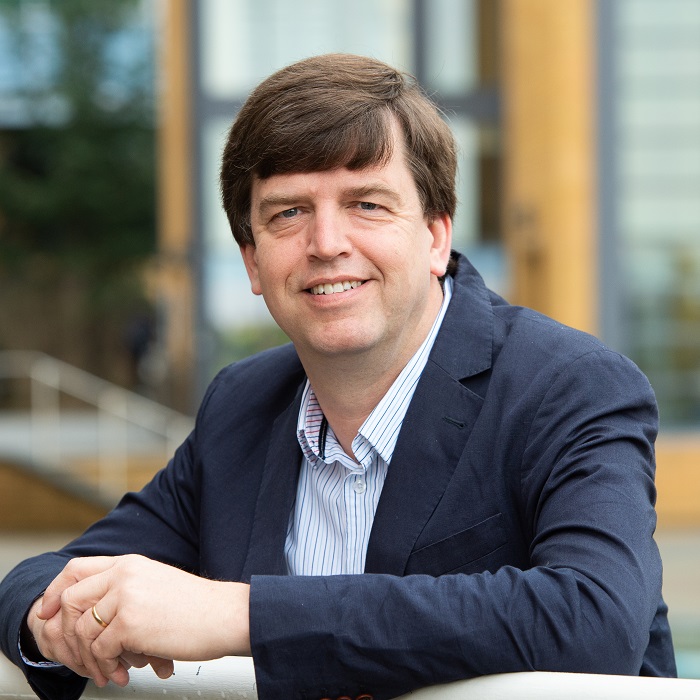
Professor Paul Sellin
Paul Sellin received his PhD in Nuclear Physics from University of Edinburgh (UK) in 1992 in the field of semiconductor nuclear detectors. His current research interests at the University of Surrey include the development and characterisation of radiation detectors and detector materials for applications in nuclear physics, medical imaging, and security detection.
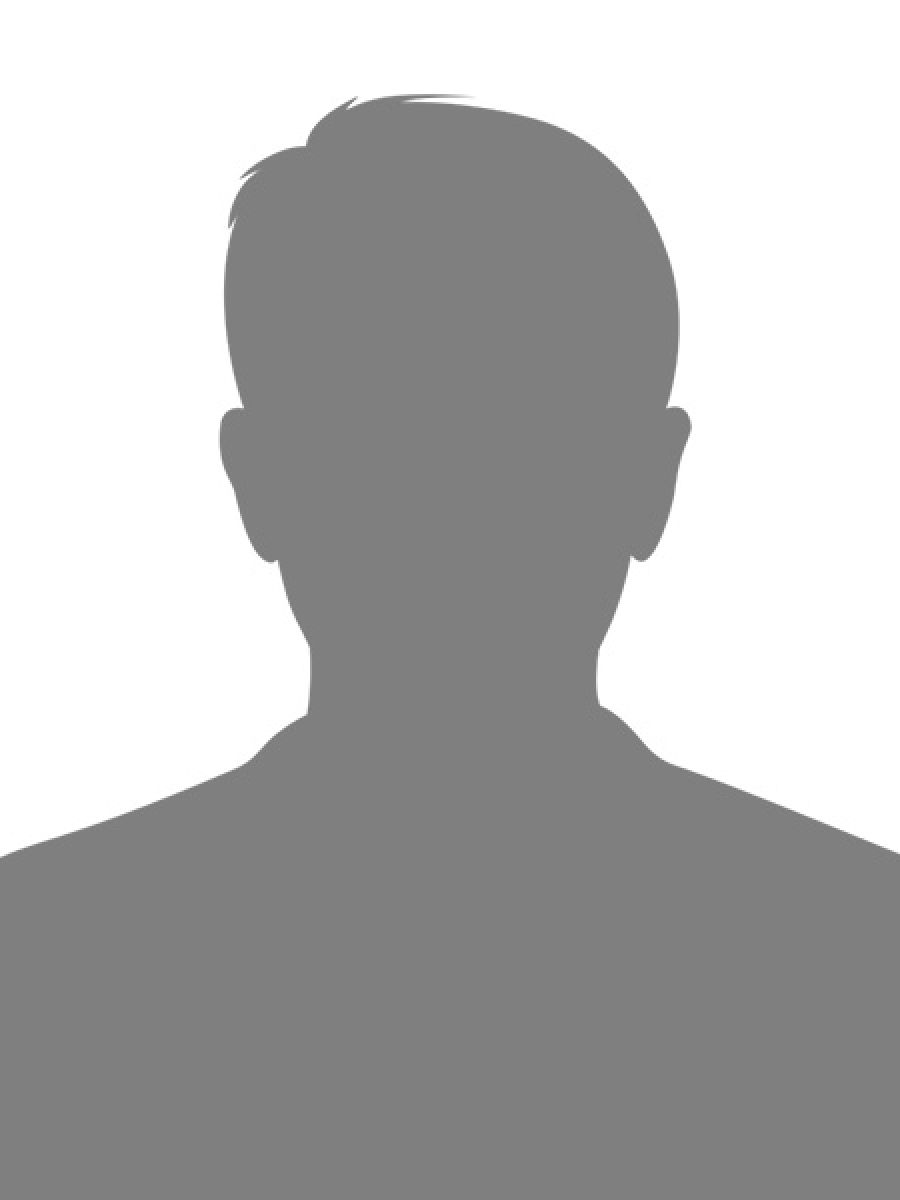
Dr Neil Gaspar
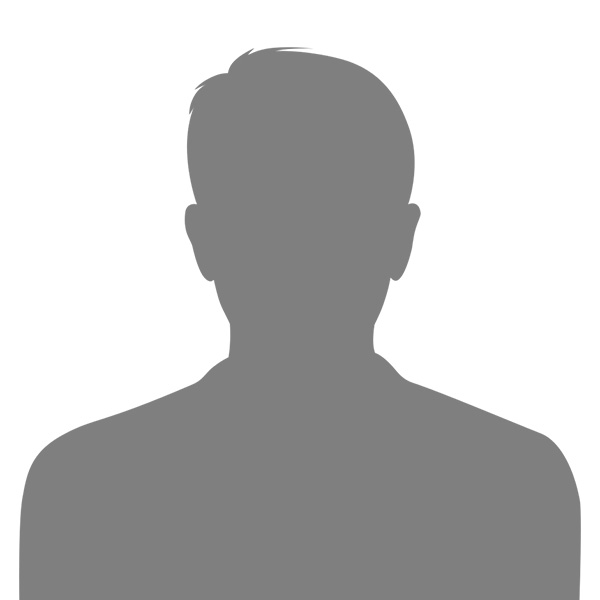
Dr Neil Gaspar
Dr Gaspar is academic programme lead for Nuclear Threat Reduction at AWE and Quantum Technology lead. His Quantum Technology work started with sensjng and algorithms. Recently he has been developing use cases and undertaking horizon scanning activities. His previous experience includes modelling the properties of materials with novel microstructure or anomalous behaviour.
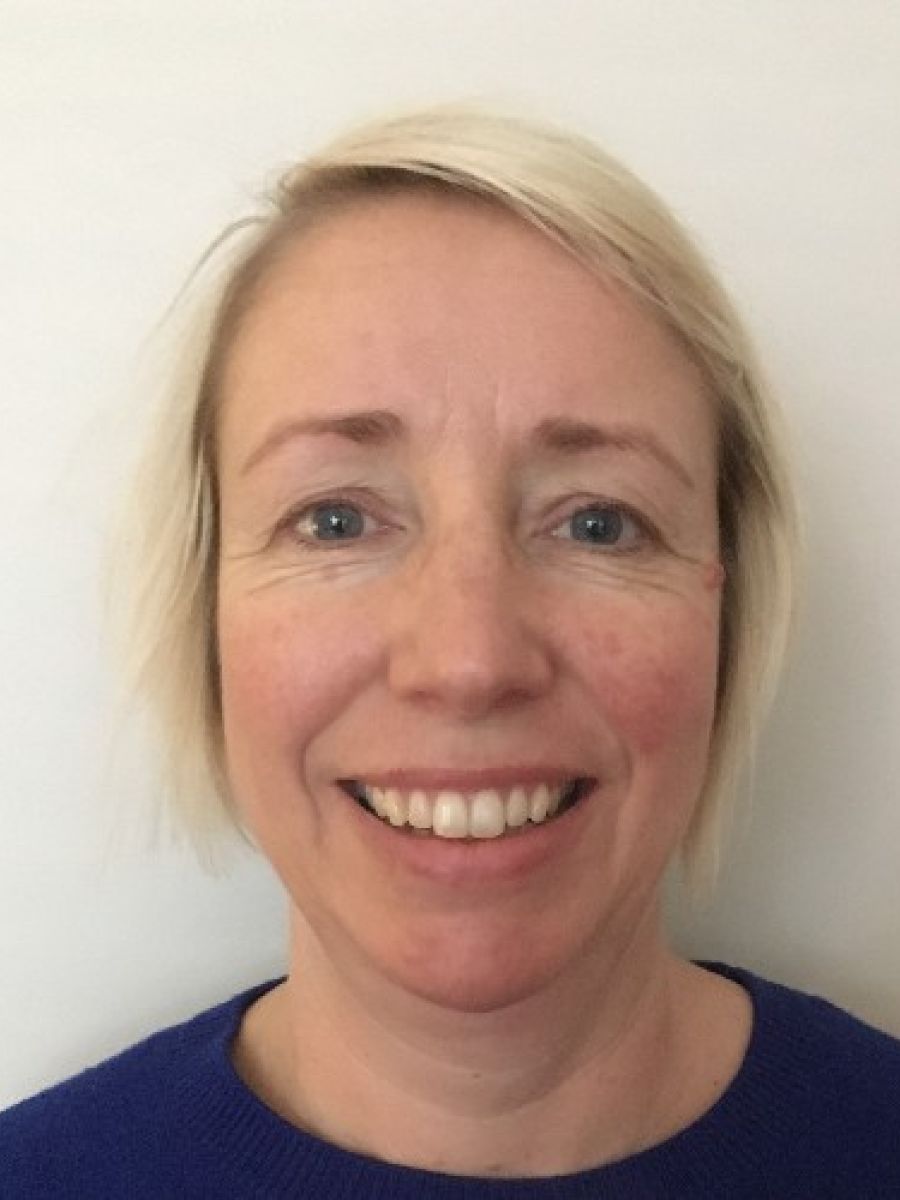
Estelle Talfan Davies
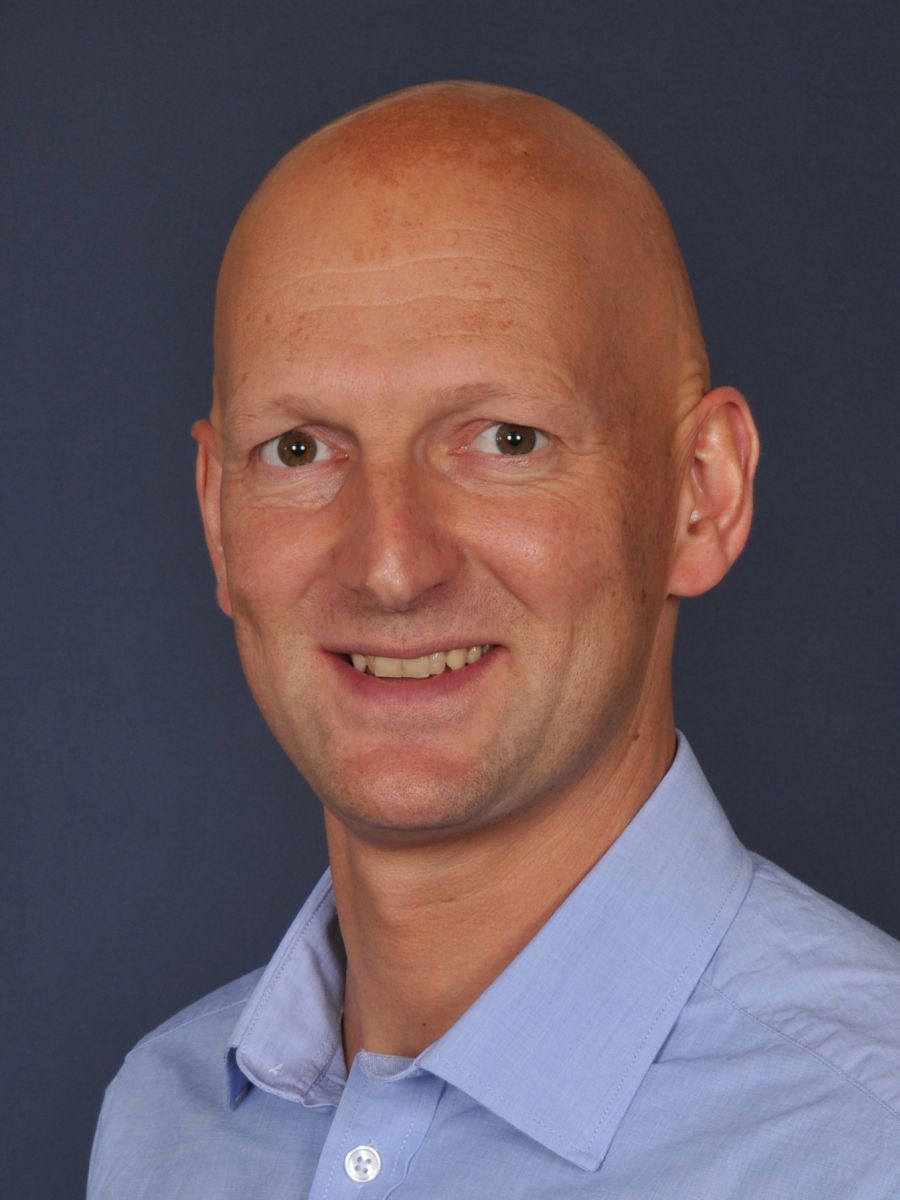
Thom Brain, Project Manager
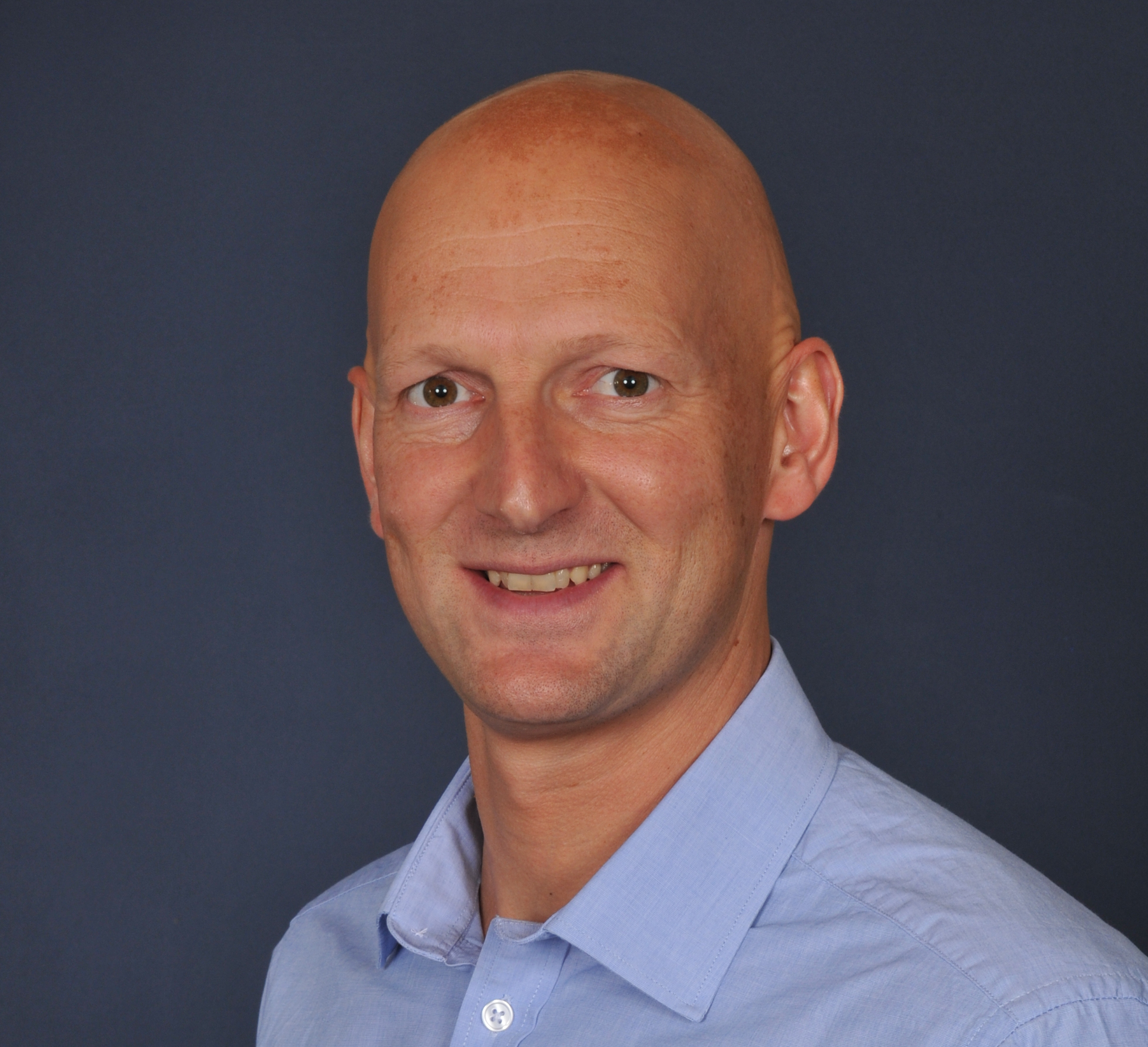
Thom Brain
Thom is the day-to-day manager of University of Bristol’s contribution to NTR-Net, including the Centre for Doctoral Partnership. He deals with funding, contracts and general administration, and is the central point of contact for enquiries.
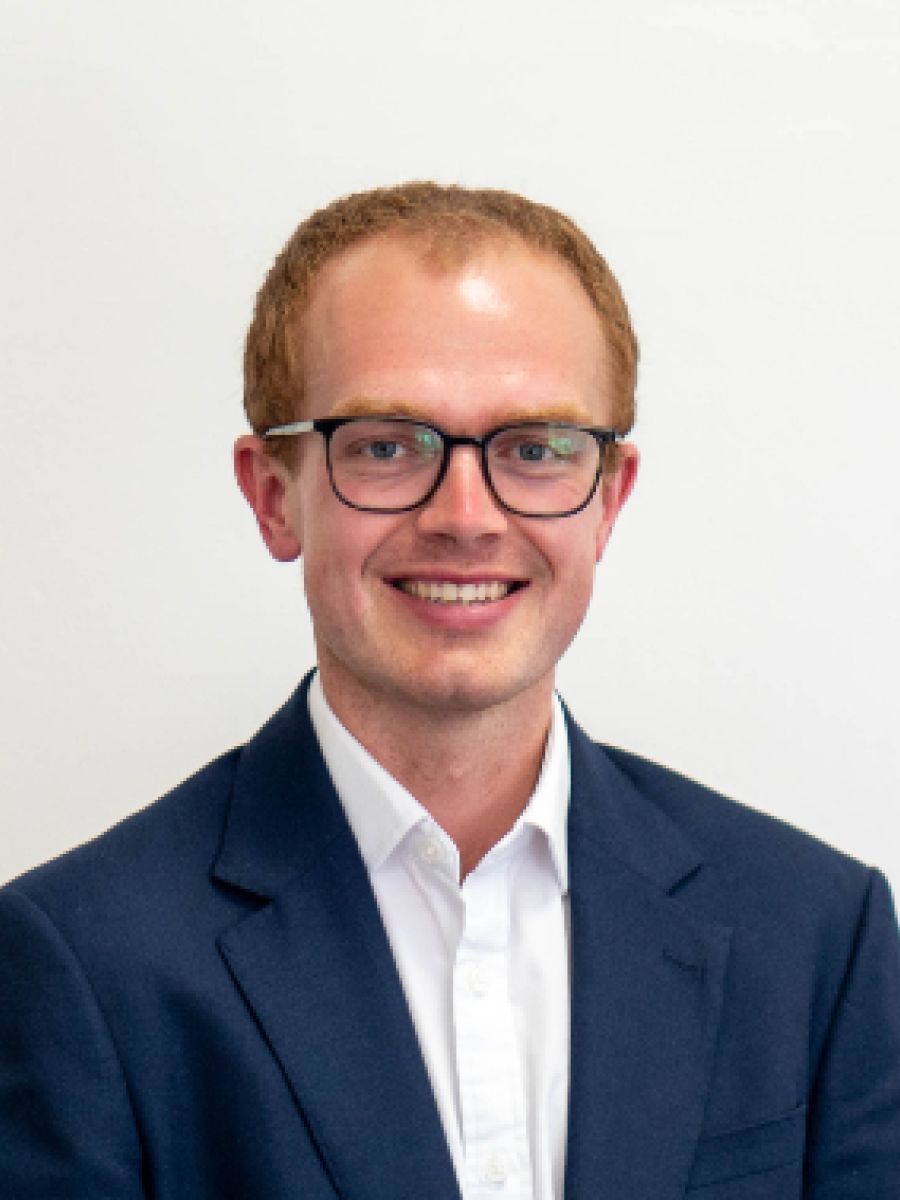
Peter Martin
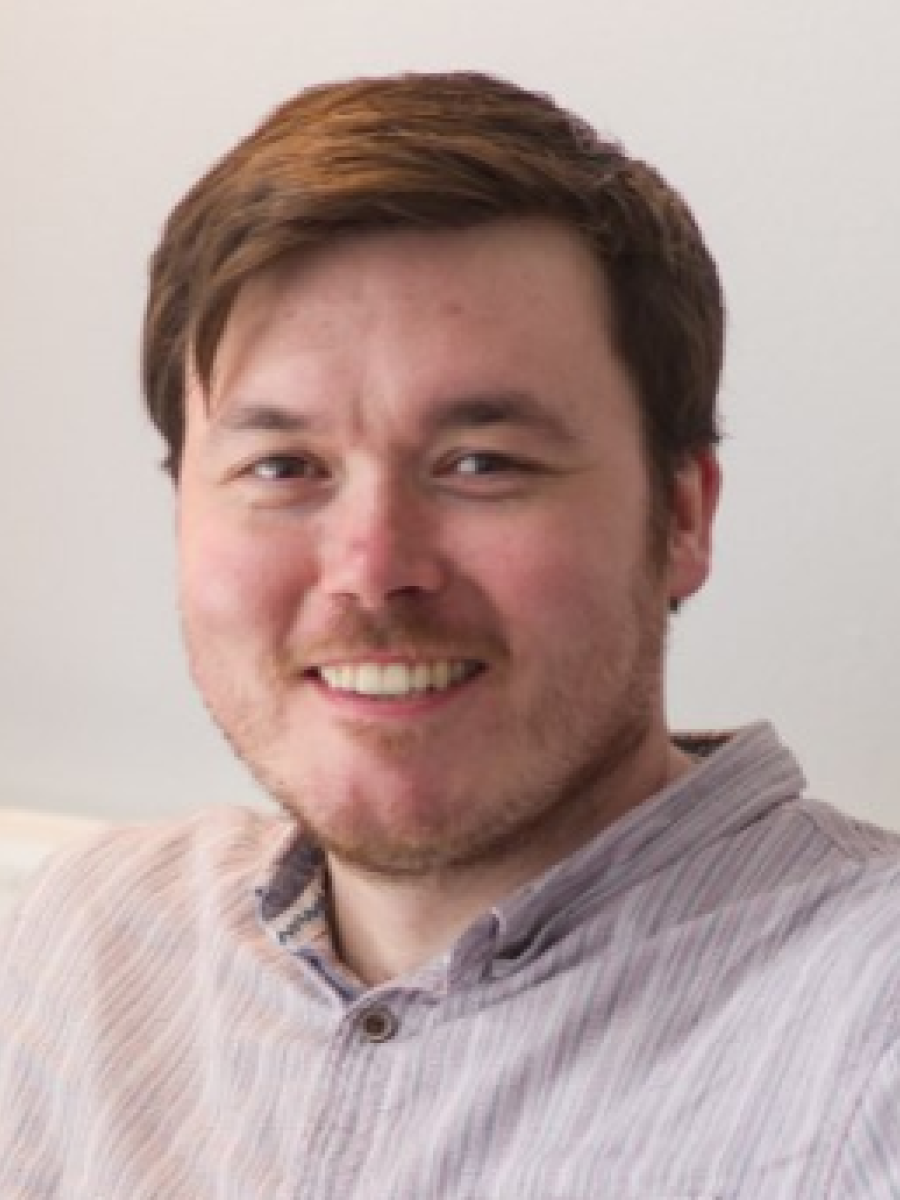
Tomas Martin

Tim Elliot

David Richards
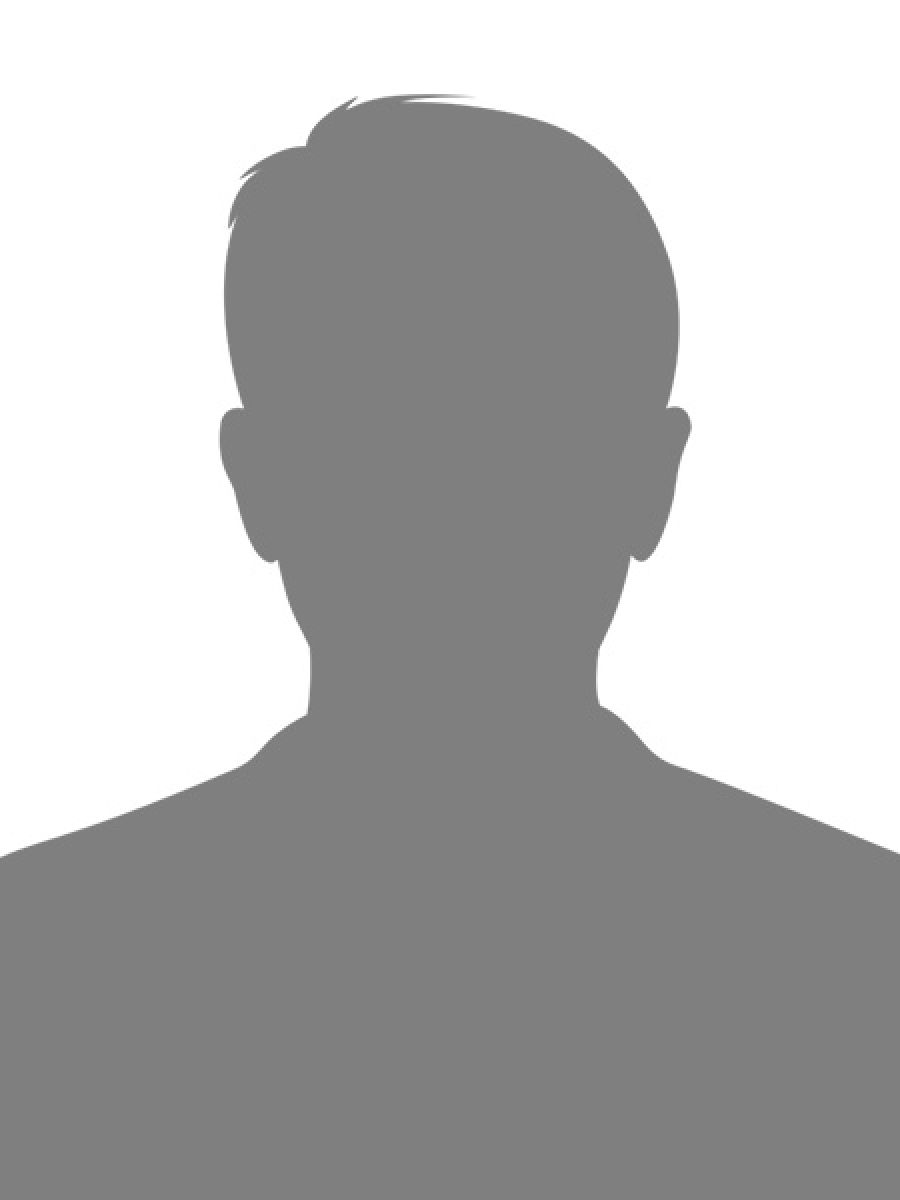